Tsetse flies in the time of climate change: What machine learning predicts
Wiida Fourie-Basson
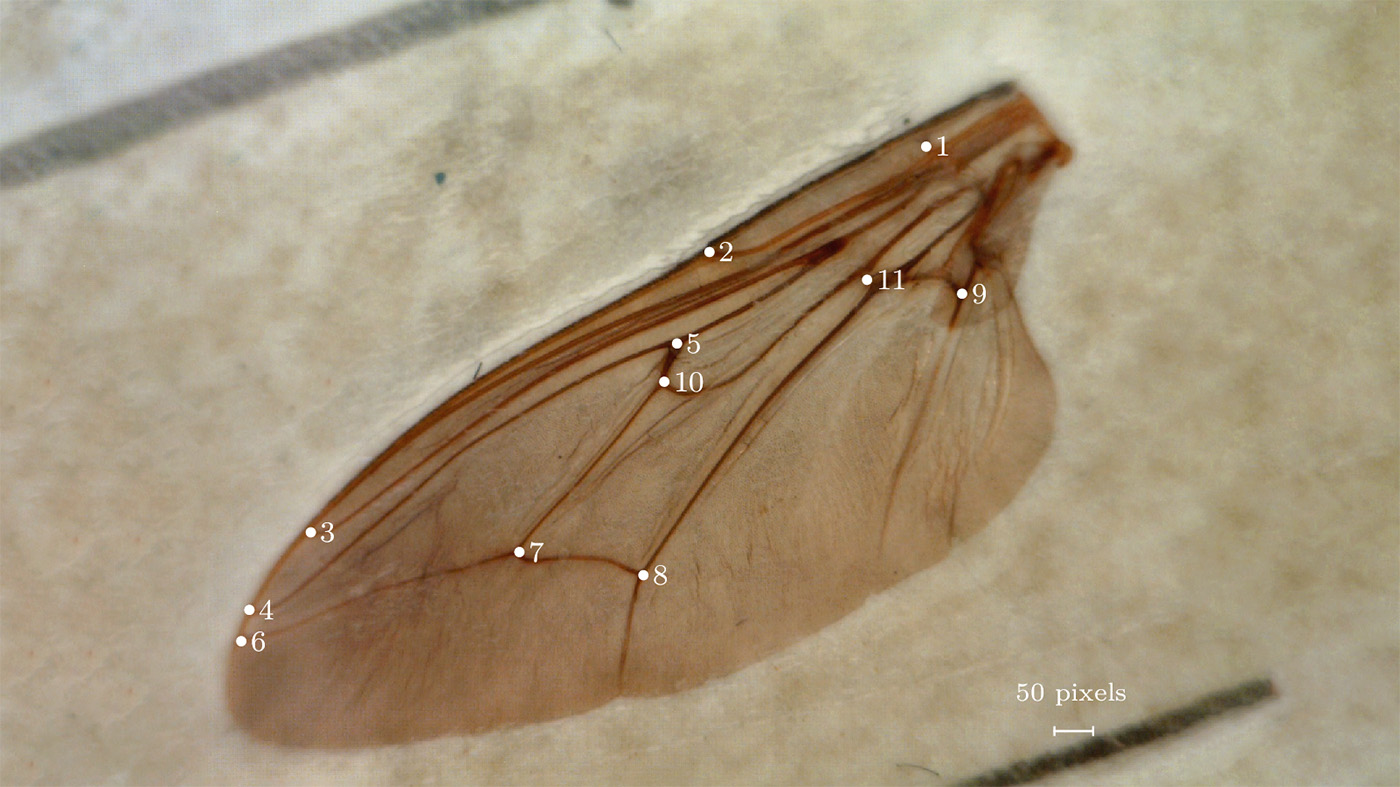
The numbered points in white on this image of a tsetse fly wing indicate 11 landmarks that may be used to compute the length, width, and various other shape measures of the wing. Manually locating the landmarks on a small subset of wings allows a machine learning model to be trained to automatically locate the landmarks on the wings of all the tsetse flies in the Rekomitjie dataset. I Image courtesy of PLOS Computational Biology
Modern machine learning methods may be key to unlocking valuable insights from a dataset of over 200 000 tsetse fly wings, collected during an 11-year study carried out at the Rekomitjie Research Station in the Zambezi Valley, Zimbabwe.
This invaluable dataset may hold the answers to what caused the dramatic collapse of tsetse fly (Glossina pallidipes) populations in the valley over the past 30 years, and whether we’ll be faced with their possible re-emergence in areas such as the Kruger National Park (KNP) in a warmer climate.
Guardian of this treasure trove of biological data is Emeritus Professor John Hargrove, founding director of the South African Centre for Epidemiological Modelling and Analysis (SACEMA), hosted at Stellenbosch University (SU).
Between 1969 and 1999, Hargrove worked as a research scientist at Rekomitjie with field biologist Dr Glyn Vale. Through his ground-breaking work on tsetse flies, Vale paved the way for the design of a prodigious number of innovative field experiments that sparked a revolution in researchers’ understanding of tsetse fly biology. While still working in Zimbabwe today, Vale is a SACEMA research associate. Together, the pair continues to analyse and publish papers based on the tsetse fly dataset.
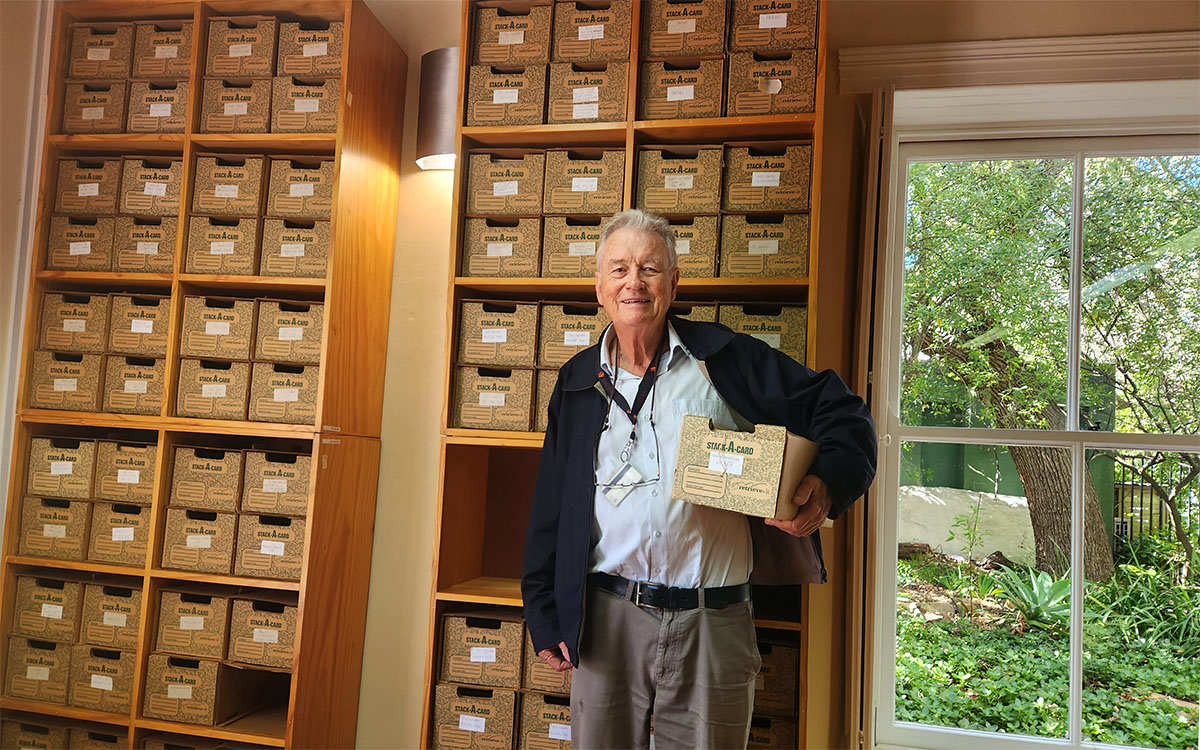
Emeritus Professor John Hargrove in front of a part of the Rekomitjie dataset stored in the director’s office at the SACEMA, hosted at SU. He custom built the bookshelves while working at Rekomitjie Research Station and brought the collection, and the book shelves, with him when he became the founding director of SACEMA . | Photo by Wiida Fourie-Basson
Why is the Rekomitjie dataset so special?
A 2020 paper describes the Rekomitjie records as “one of the most comprehensive longitudinal datasets of tsetse fly count data available to date’’. Apart from the over 200 000 tsetse fly wings, it also contains ovarian dissection data from more than 180 000 female tsetse flies, nutritional data from 40 000 tsetse flies, and catches from more than 10 sampling systems used since 1960. There are also valuable temperature, humidity, and rainfall records, as well as other meteorological data, collected from the same area since 1959.
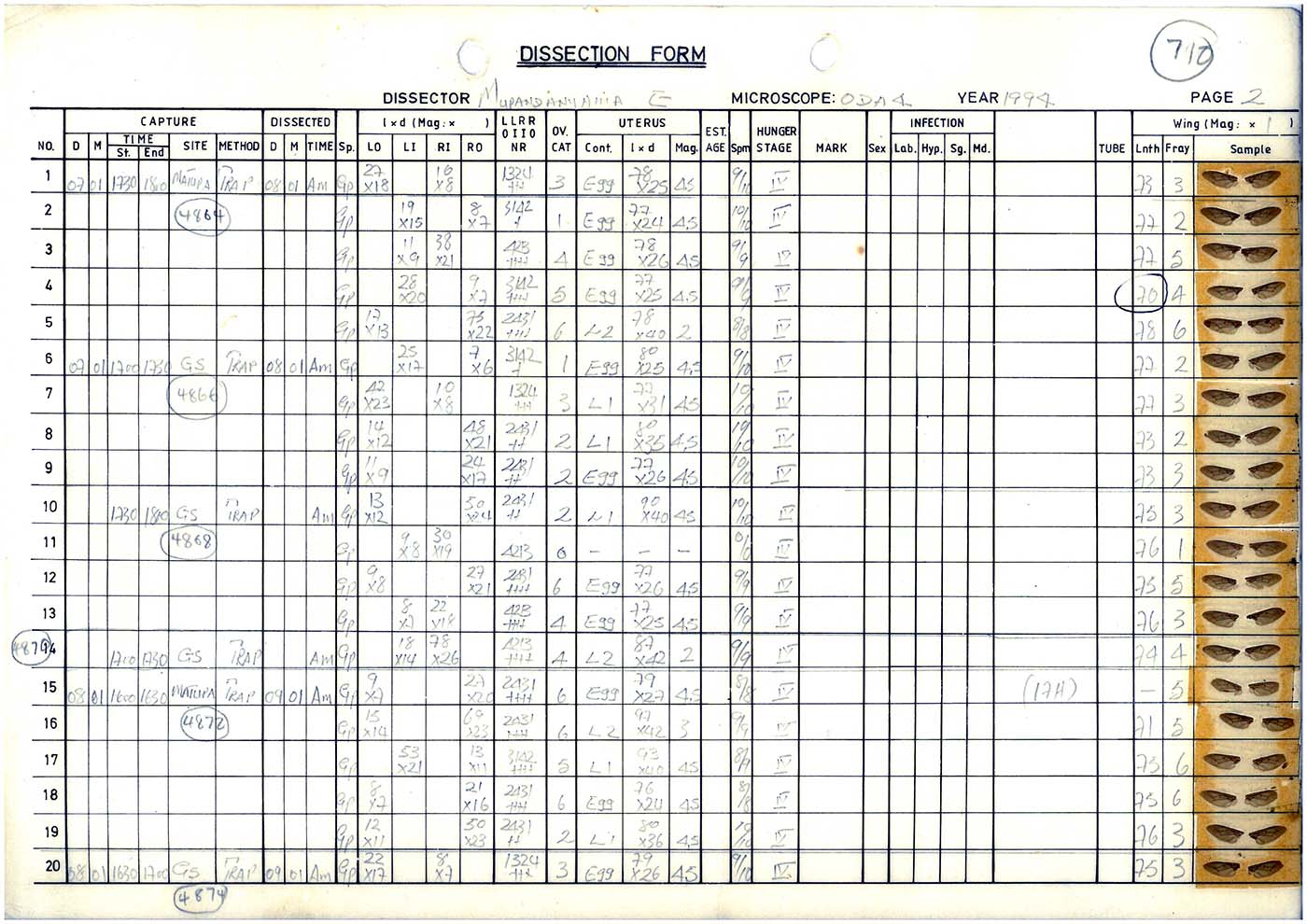
A typical dissection form from the Rekomitjie dataset, containing detailed information on each tsetse fly caught, from the date, time, place, and method of the catch, to the name of the dissector and the results of the dissection. The latter include infection status, estimated age, length, and nutritional status. | Source: Rekomitjie dataset
So, what can the Rekomitjie dataset tell us about what to expect from a warmer climate?
Unlike mammals and birds, insects such as tsetse flies cannot regulate their own body temperature. As such, their rates of development and mortality are strongly influenced by environmental temperatures. Pupae cannot survive at sustained temperatures below 16 °C or above 32 °C.
A 2018 study, based on 27 years of data from Rekomitjie, suggests that temperature increases over the last three decades have already caused major declines in tsetse fly populations in those areas where they traditionally occurred. The study was based on laboratory and field measures of fly densities taken since the 1990s, and nearly continuous records of climatic data collected since 1975 by the Rekomitjie researchers.
Catches of tsetse flies from cattle in Zimbabwe’s Mana Pools National Park have declined from more than 50 flies per animal per catching session in 1990, to fewer than one fly per 10 catching sessions in 2017. Since 1975, mean daily temperatures in the study area have risen by nearly 1 °C. In the hottest month, November, this number is almost double.
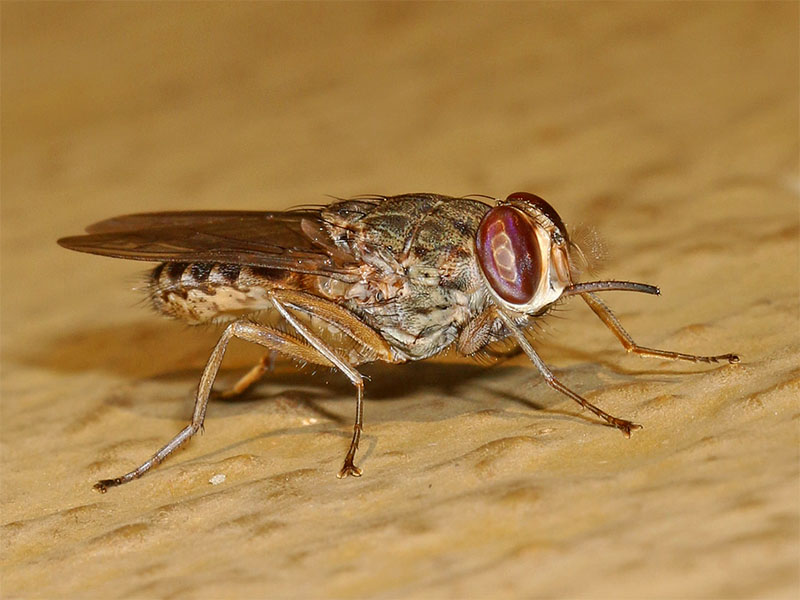
Tsetse flies are blood-feeding insects that transmit trypanosome pathogens, which cause sleeping sickness in humans across sub-Saharan Africa. Without treatment, the disease is fatal. In livestock, parasites of this genus cause nagana (from a Zulu word meaning ‘low or depressed in spirit’). | Photo by Judy Gallagher
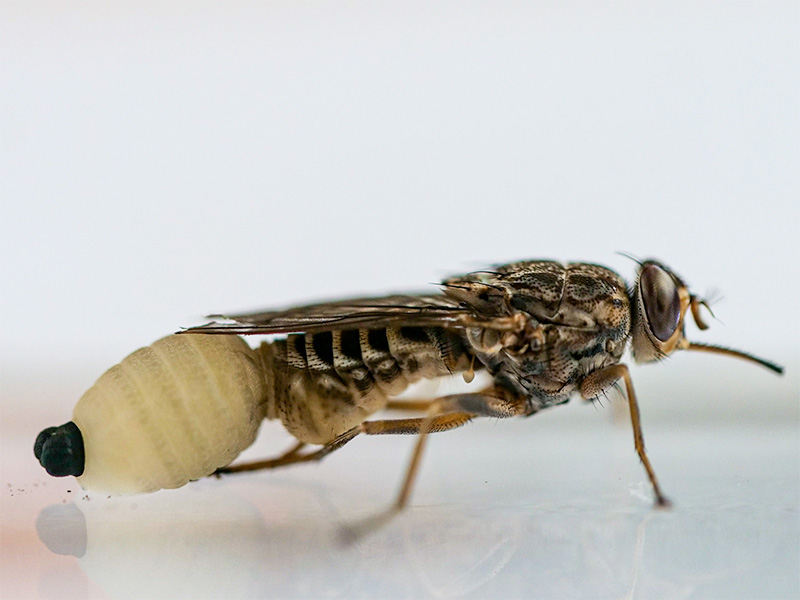
Tsetse flies have a slow reproductive cycle. After ovulation, it takes eight to 15 days for a single larva to develop in the mother. After the larva is deposited, it buries itself in the ground for 20 to 40 days before it emerges as an adult. | Photo by Daniel Hargrove
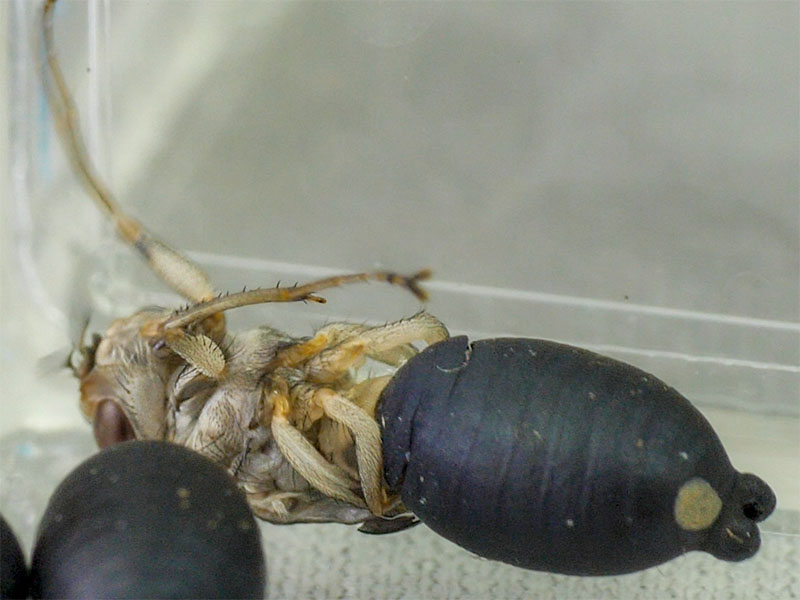
An intriguing fact about tsetse flies’ reproductive biology is that the larvae, deposited in soil, mature within eight days for the flies to emerge as adults. Importantly, immediately after the wings expand and harden, their length does not change. | Photo by Daniel Hargrove
He regards the 2018 study mentioned above as one of the first to indicate a link between higher temperatures and an increased susceptibility in certain African regions to sleeping sickness (African trypanosomiasis), transmitted to humans by infected tsetse flies.
Both the Hwange National Park in Zimbabwe and the KNP in South Africa are examples of large conservation areas in which suitable hosts and habitats for tsetse flies abound, Hargrove warns. “Tsetse did occur in these areas in the 19th century, but their numbers were always marginal because the winters were rather too cold. With the massive rinderpest outbreak of the middle 1890s, when the vast majority of ungulates [hoofed animals] died, tsetse disappeared from these areas and have never re-established themselves again. But, if temperatures continue to increase, there is a danger that they may re-emerge.”
To date, however, no one has had sufficient data to model the risks of such an eventuality.
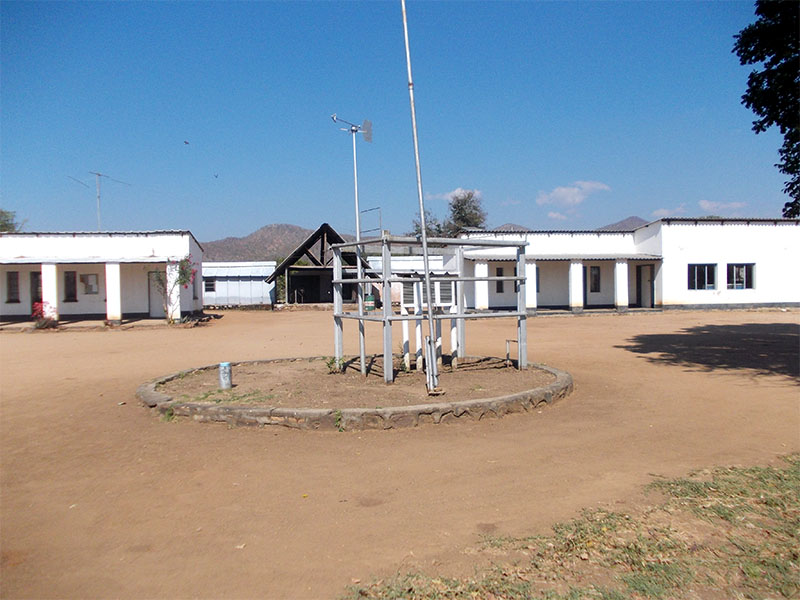
Work at the Rekomitjie Research Station in Zimbabwe has highlighted the central importance of temperature in tsetse fly population dynamics. The research station is located inside Mana Pools National Park, a protected area that has been free of agricultural activities since 1958. In 1984, the park was designated a UNESCO World Heritage Site. | Photo by Prof John Hargrove
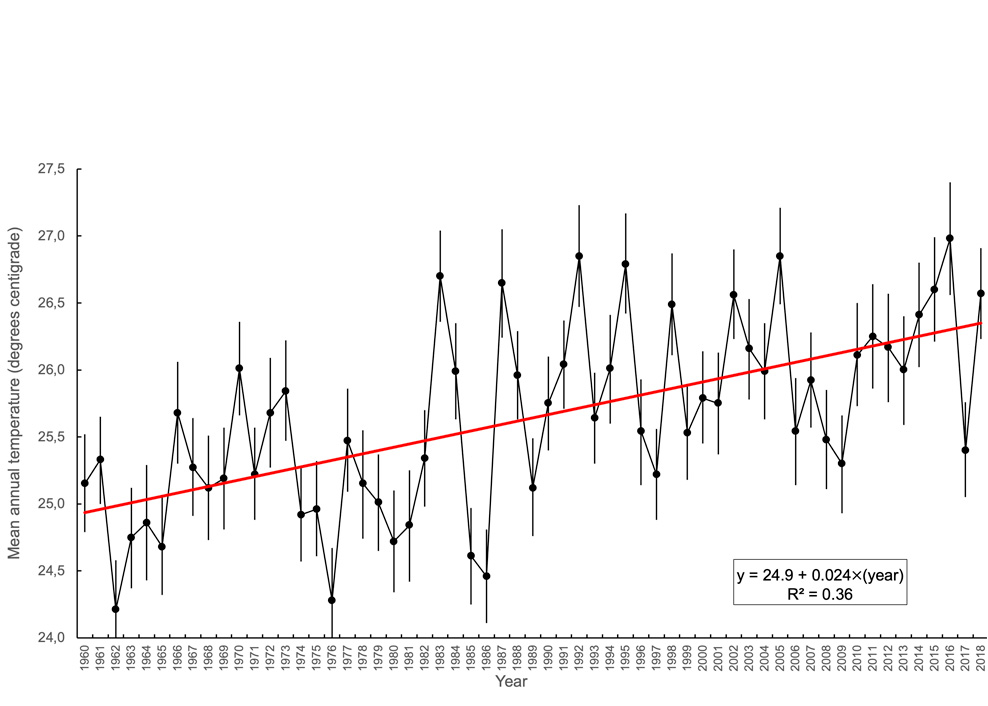
Mean annual temperatures at the Rekomitjie Research Station have increased by 0.024 °C per year since 1960 — a total of 1.4 °C over the 58 years until 2018. I Graph generated by Prof John Hargrove
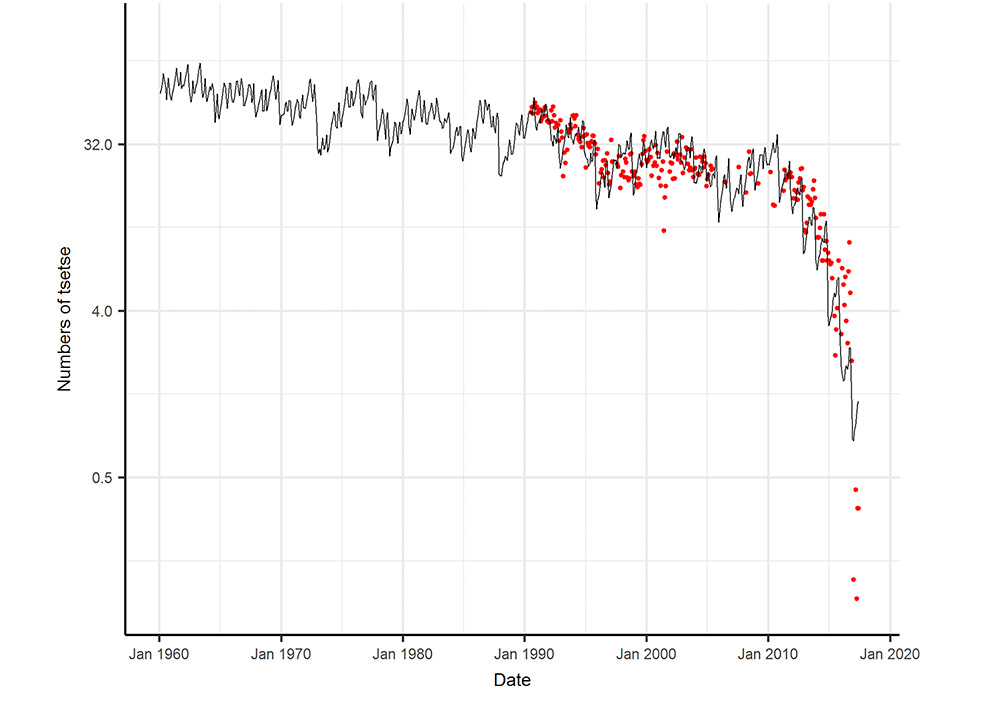
Since the 1960s, wild tsetse flies have been caught regularly from cattle for insecticide tests conducted at Rekomitjie Research Station. Before 1990, catches averaged more than 50 flies per animal per afternoon; in 2017, teams frequently failed to catch a single fly in a session. I Source: Rekomitjie dataset
Bringing the Rekomitjie dataset into the 21st century
In 2017, with one of the 27 boxes of valuable tsetse fly data under his arm, Hargrove approached machine learning experts in the Applied Mathematics Division at SU. His objective: automating the measurement of wing shape in tsetse flies.
But why focus on wing shape? To date, researchers have been unable to ascertain whether variations in tsetse flies’ wing shape and size are attributable purely to genetic differences between populations, or whether environmental factors also play a role.
Questions such as this can only be answered on grounds of a large sample size representing several seasons. While SACEMA holds by far the largest collection of tsetse fly wings ever amassed, it is, however, extremely time consuming to manually measure small, fragile things such as insect wings. As a result, previous studies have been limited to sample sizes of a few hundred wings at most.
This is where machine learning comes in. This branch of artificial intelligence enables systems to extract patterns and dependencies from data without being explicitly instructed on how to do so. Applied mathematicians often use it for modelling purposes, which is exactly how the data locked away in those 27 boxes was exploited. The exact process consisted of a number of steps.
Step 1: Photograph the tsetse fly wings with a high-resolution microscope camera. Once the wings have been digitised and numbered, capture the rest of the data associated with each pair in an Excel spreadsheet. This includes the date, time, site, and method of the catch, the name of the dissector, and the results of the dissection, including infection status, estimated age, length, and nutritional status.
To date, Dr Pietro Landi, Prof Cang Hui, and their students in SU’s Department of Mathematical Sciences have photographed about 90 000 pairs of wings from 13 of the 27 boxes.
Step 2: Train a machine learning model to automatically locate 11 anatomical landmarks in any given wing image. For the purposes of machine training data, Prof Willie Brink from the Applied Mathematics Division built a custom annotation tool that allowed him to manually pinpoint landmark positions on 2 000 wing images, all in just a few hours. This initial phase of manual categorisation is vital to the accuracy of the end product: “A model is only as good as the quality of data it is trained on. If I do 1% of the work well, we can train a model to do the remaining 99%,” Brink explains.
To this end, Brink and two of his MSc students, Shane Josias and Mulanga Makhubele, experimented with a number of deep learning architectures.
Step 3: Find postgraduate students to perform a morphometric analysis of tsetse fly wings by quantitatively analysing their form and shape. This would involve studying how the wing shape of tsetse flies changes between males and females, over time, and with the change of seasons.
Then MSc student Dylan Geldenhuys first became involved with the Rekomitjie dataset project when he volunteered to take digital photos of the tsetse fly wings for his graduate student project. This led to a growing interest in the morphometry of the wings — extracting certain features would, he hoped, help machine learning models expose how this morphometry reacts to changes in weather and climate.
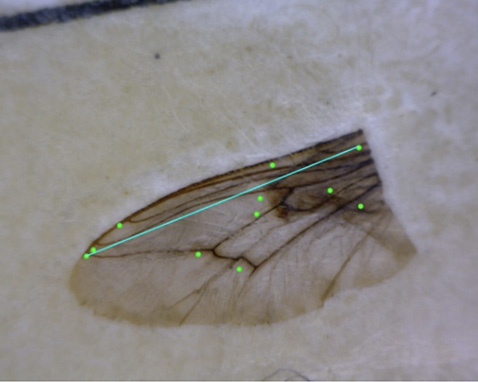
1. Missing end
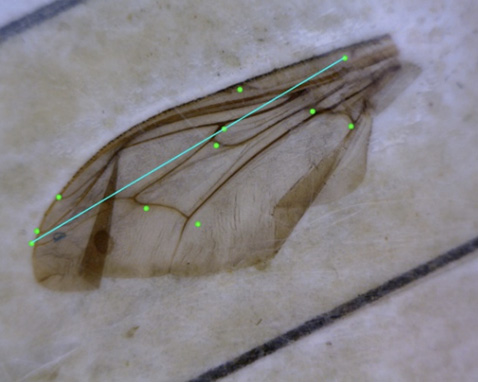
2. Folded wing
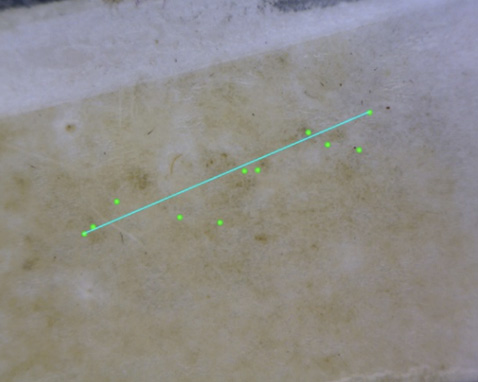
3. Missing wing
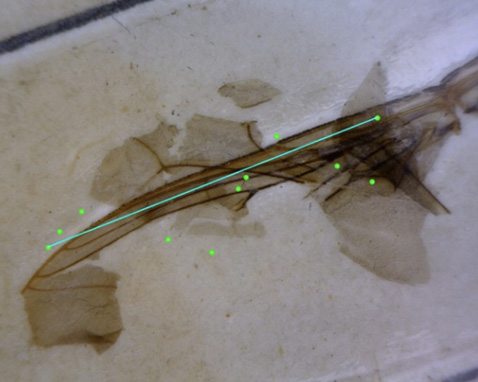
4. Badly damaged wing
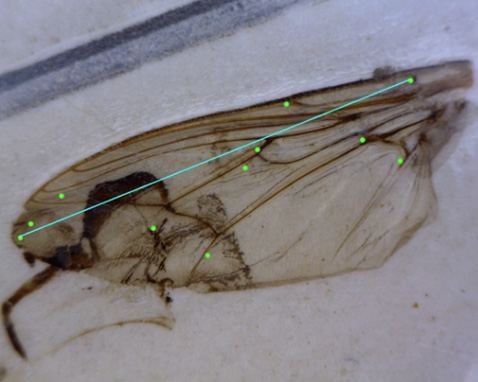
5. Major artefacts
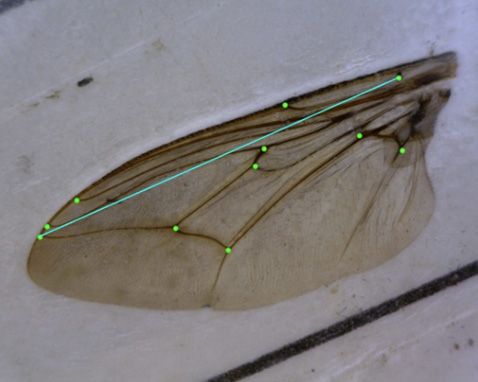
6. Complete wing
Deep learning approaches to landmark detection in tsetse wing images. I Images courtesy of PLOS Computational Biology
For his MSc in applied mathematics, with Hargrove, Dr Marijn Hazelbag, and Jeremy Bingham as study leaders, Geldenhuys used deep learning approaches to detect and accurately position identifiable landmarks on images of tsetse fly wings. Using more than 28 000 images of tsetse wings, he developed a landmark dataset that can be employed in future morphometric analysis of tsetse fly wings, and potentially as a starting point for studies on the wings of other insect species, particularly those that transmit diseases to humans, livestock, and plants.
In practice, modellers can use this dataset to distinguish between populations of tsetse flies from different geographical areas, based on differences in wing shape. The objective is to be able to locate ‘biological islands’ of tsetse fly distribution so that they may be targeted for control.
At present, BScHons student Nuhr Ryklief is investigating whether Geldenhuys’ landmark dataset can be extended across the full set of photographed wings. He is also working on the morphometric analysis of changes in wing shape over time.
Another BScHons student, Leandru Fleidl, is using the Rekomitjie dataset to quantify the predicted effects of climate change on the future distribution of tsetse fly populations, and to determine whether the flies are likely to pose a threat to animals and people in the KNP. Predictive models such as this one can help scientists understand where and how quickly, under changing climatic conditions, tsetse fly populations are likely to increase in number and also spread.
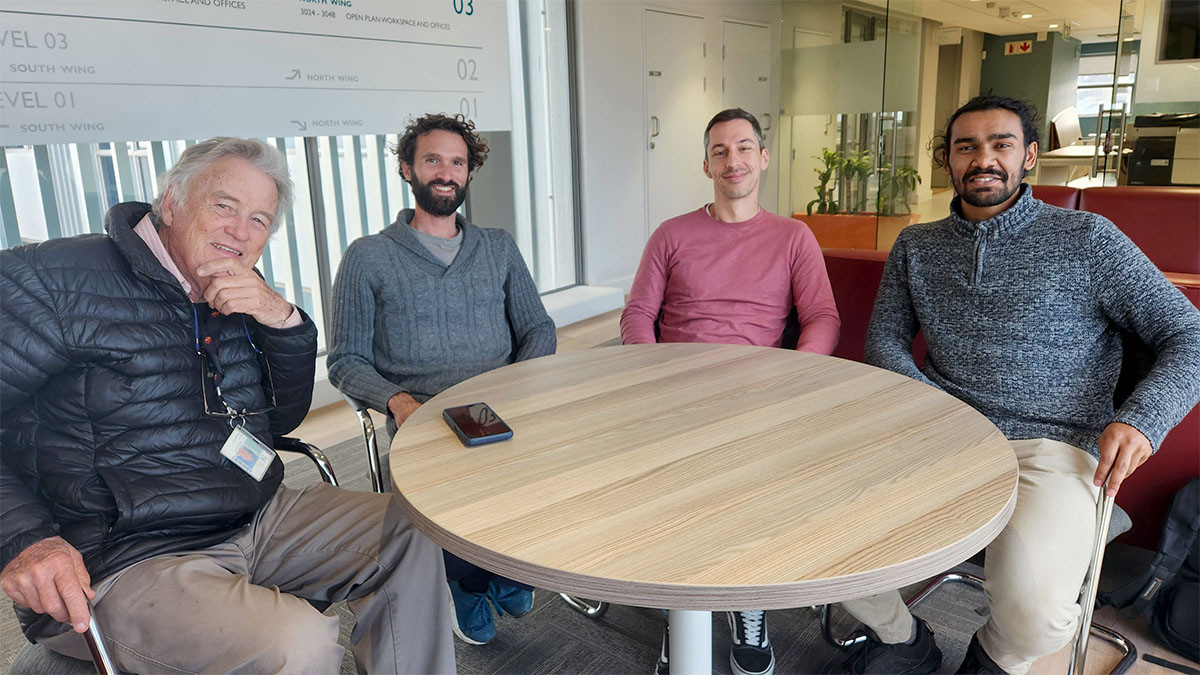
Discussing the progress of his research project with BSc Honours student Nuhr Ryklief (on the far right) are his study leaders (from left to right) Prof John Hargrove, Dr Pietro Landi, and Prof Willie Brink. | Photo by Wiida Fourie-Basson
Safeguarding the Rekomitjie dataset for the future
“It is crucial that all the data from the Rekomitjie Research Station is archived — with the fullest and clearest possible notation — to ensure that it will be of optimal use to future generations of biologists”, says Hargrove.
So far, the team has barely scratched the surface. Only two of the 27 boxes (14 000 of 205 000 pairs of wings) have been fully digitised.
According to Hargrove, the collection holds enough data to support at least 50 doctoral theses on subjects ranging from birth and ageing processes, physiology, and insect demography to population dynamics, mortality, extinction probabilities, and much more, not to mention the effects of meteorological changes on all of these topics.
“It is fair to say that we have more high-quality field information available on tsetse than anybody has on any other insect species on the planet. This information could, and should, be used to inform studies on other insects.
“If the Rekomitjie data were to be lost, it could never be reproduced. It would be lost, to everybody, for all of time,” says Hargrove.
Climate change, disease, and human health
- By the end of the century, global temperatures are expected to have risen by 3–5 °C. This will affect all infectious diseases, especially those spread by insects (vector-borne diseases).
- It is crucial to both understand and have the ability to predict the effects of climate change on vector-borne diseases and pathogens — something that relies heavily on modelling.
- Climate-related changes in the dynamics between hosts, vectors, and parasites impact the well-being, public health, and economy of African communities.
- Climate change is expected to alter the way in which diseases spread in Africa. Vector-borne ones such as malaria, dengue, Chikungunya, Rift Valley fever, Zika, and sleeping sickness are all predicted to spike in prevalence in the coming decade.
- Recent research indicates that while temperature increases might lead to faster development in the immature stages of tsetse flies’ life cycle, they may also decrease overall lifespan in adult flies, creating a complex scenario for transmission dynamics — a topic that requires further research.
More about tsetse flies and sleeping sickness
- African trypanosomiasis, also known as ‘sleeping sickness’, is a deadly disease spread by tsetse flies in sub-Saharan Africa. These flies transmit parasites (protozoa of the genus Trypanosoma) that cause severe illness in humans and animals. Without treatment, sleeping sickness is usually fatal.
- Every year, across Africa, sleeping sickness is responsible for the loss of approximately 202 000 disability-adjusted life years — the number of years lost due to ill health, disability, or early death.
- The disease is endemic in 36 sub-Saharan African countries. The Democratic Republic of the Congo has the highest burden, having been host to approximately 61% of all reported cases in the past decade.
- Most exposed people live in rural areas and depend on agriculture, fishing, animal husbandry, or hunting for a living.
- Tsetse fly populations are highly resilient. They can persist at very low densities and are highly mobile. The 29 species and subspecies are also widely dispersed.
- As such, eradicating tsetse flies from an area is not only exceedingly difficult, but even if it is achieved, the area remains susceptible to re-infestation from neighbouring populations.
Sources: World Health Organization and the World Organisation for Animal Health
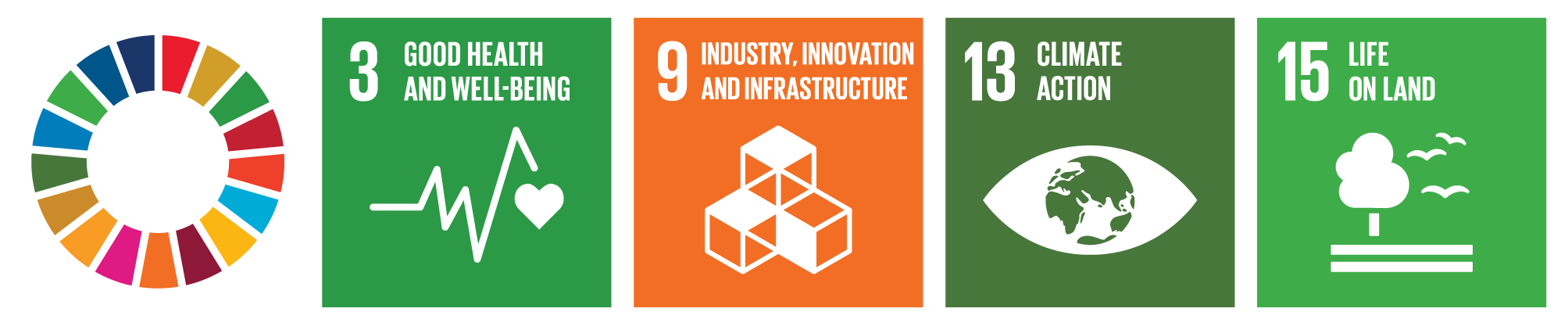
The research initiatives reported on above are geared towards addressing the United Nations’ Sustainable Development Goals numbers 3, 9, 13, and 15, and goals number 3, 5, and 7 of the African Union’s Agenda 2063.
Useful links
South African Centre for Epidemiological Modelling and Analysis (SACEMA)
SU’s Division of Applied Mathematics
What changes in temperature mean for Africa’s tsetse fly
Zambezi Valley may soon be too hot for tsetse flies
In parched Zimbabwe, climate change pushes tsetse to new areas
Climate change research to prevent sleeping sickness outbreaks in Tanzania
x (formerly Twitter): @scienceSUN, @MatiesResearchtsetse and @StellenboschUni