By Atman Prins
Accurate crop type maps are important for obtaining agricultural statistics such as water use or harvest estimations. The traditional approach to obtaining maps of cultivated fields is by manually digitising the fields from satellite or aerial imagery. However, manual digitising is time-consuming, expensive and subject to human error. Automated remote sensing methods have been a popular alternative for crop type map creation, with machine learning classification algorithms gaining popularity for classifying crop types from satellite imagery. However, using light detection and ranging (LiDAR) data for crop type mapping has not been widely researched.
This study evaluates the use of LiDAR data and machine learning algorithms for mapping vineyards. Vineyards are planted in rows spaced at various distances, which can cause spectral mixing within individual pixels and complicate image classification. Four resolution where used for generating normalized digital surface model and intensity derivatives from the LiDAR data. In addition, texture measures with window sizes of 3×3 and 5×5 were generated from the LiDAR derivatives. The different combinations of the resolutions and window sizes resulted in eight data sets that were used as input to 11 machine learning algorithms. A larger window size was found to improve the overall accuracy for all the classifier–resolution combinations. The results showed that random forest with texture measures generated at a 5×5 window size outperformed the other experiments, regardless of the resolution used. We conclude that the random forest algorithm used on LiDAR derivatives with a resolution of 1.5m and a window size of 5×5 is the recommend configuration for vineyard mapping using LiDAR data.
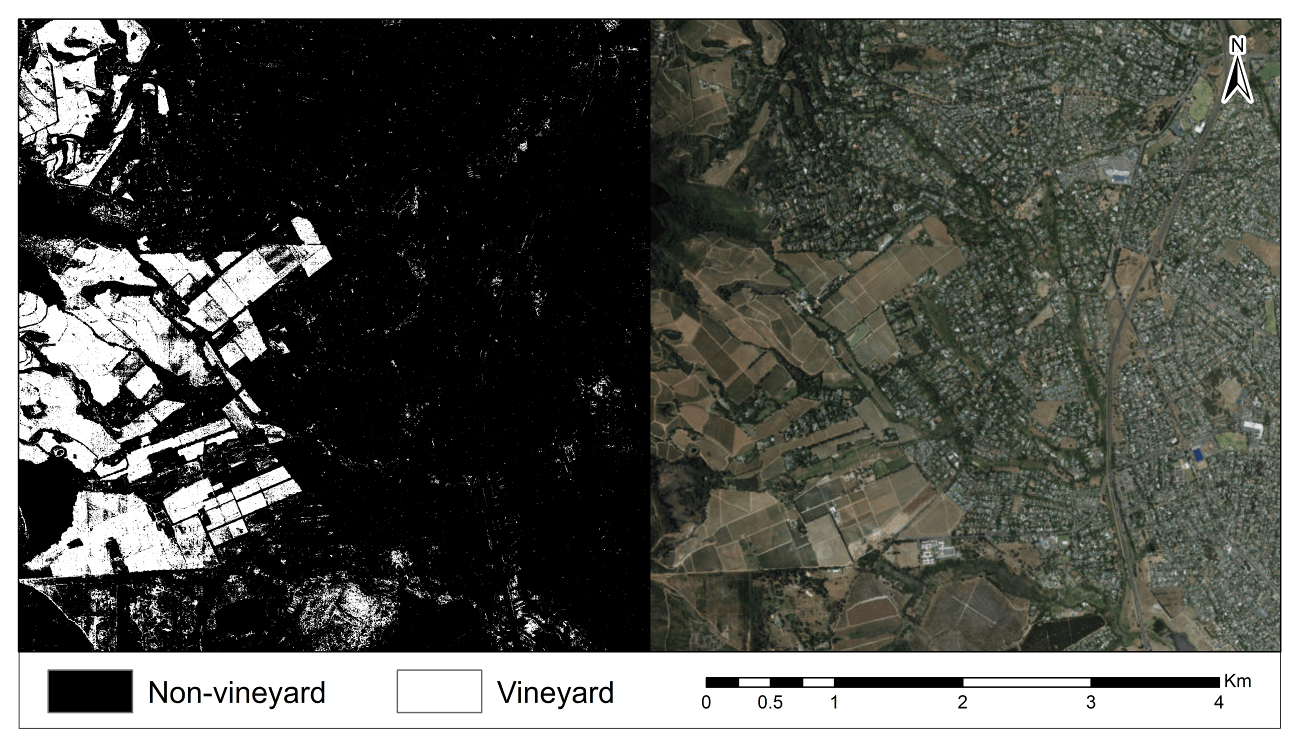
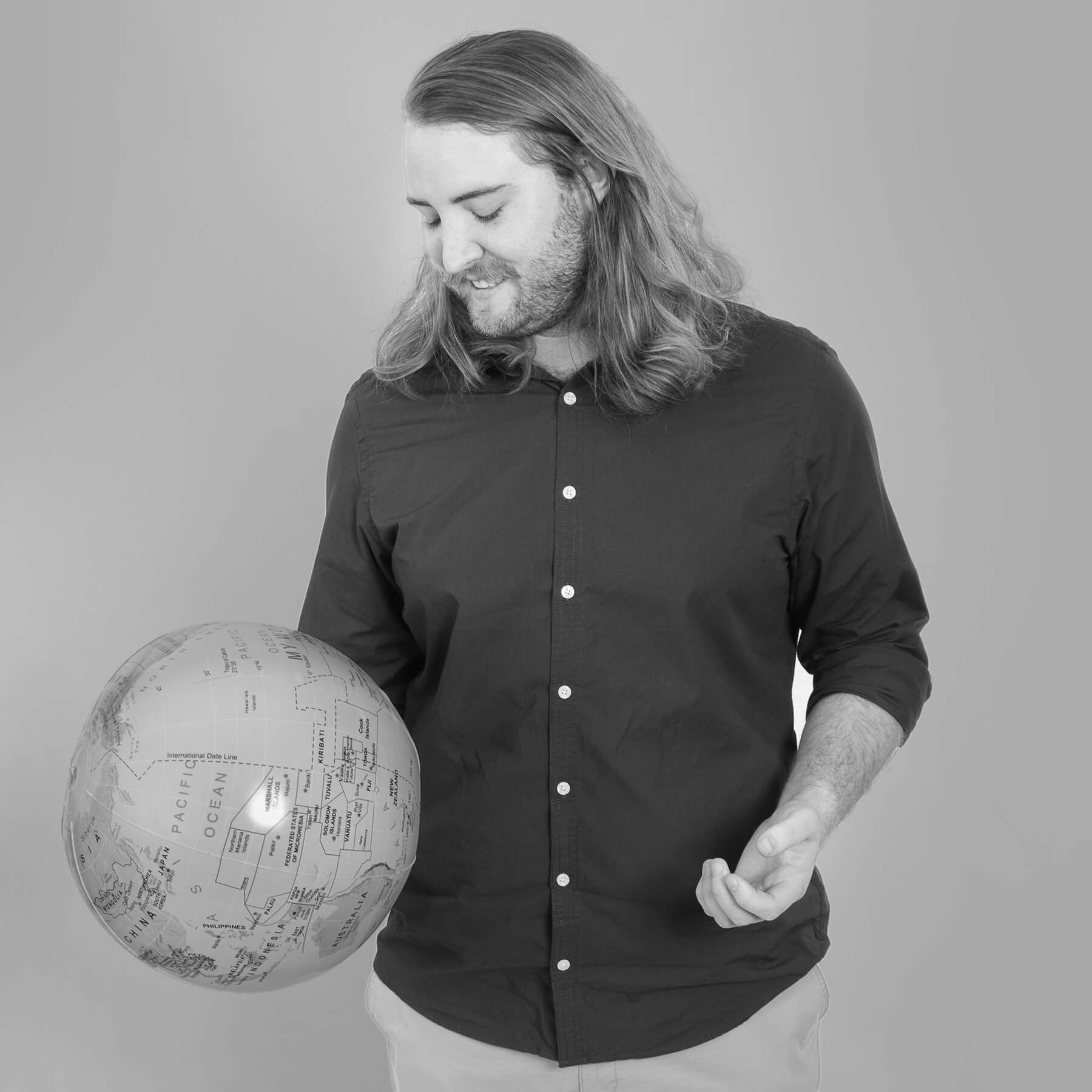
The above is the extract from the research paper by Atman Prins as part of his Msc at Stellenbosch University. The full article can be found here.
Atman completed his Bsc Honours in Geoinformatics in 2016, with his research focusing on using LiDAR data, template matching and region growing in order to count trees in a forestry plantation. In 2017 he started with his Msc in Geoinformatics and continued on with his research using LiDAR data. However, for his Msc research he focused on classifying crops using machine learning, LiDAR data and data fusion with spectral data. While he was busy with his MSc, he was employed part time at the CGA. In 2019 he was employed as a full-time GIS & EO analyst while finishing his MSc.