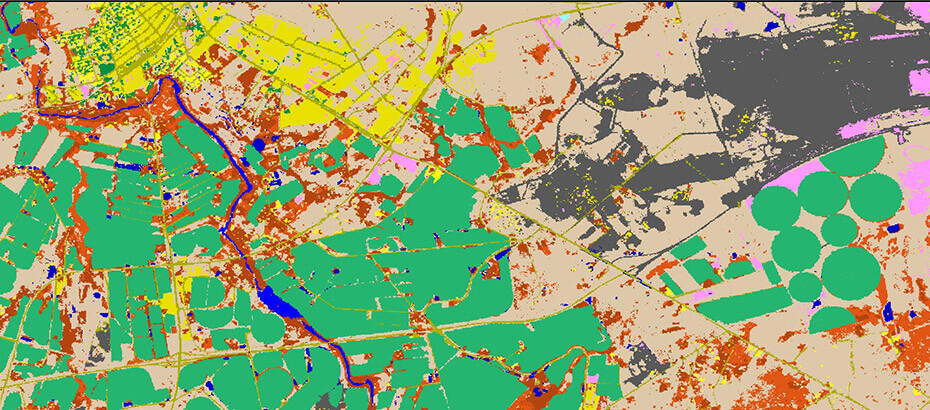
For geographical information to be most effective in decision making it must be detailed, up-to-date and accurate (Benz et al. 2004; Carvalho et al. 2004). This also applies to land cover data, which is essential for decisions related to land evaluation (Kalogirou 2002; Van Niekerk 2008), urban and regional planning (Tapiador & Casanova 2003; Culshaw et al. 2006) and environmental management (Lee et al. 2001; Phua & Minowa 2005). According to (Lui et al. 2002), timely and accurate land cover maps are required to understand the relationships and interactions between human and natural phenomena. Regularly-updated land cover maps are also essential in assessing and monitoring ecological resources and to formulate appropriate policies (Blaschke et al. 2005).
Satellite remote sensing is frequently used to produce land cover maps for large areas such as South Africa (Campbell 2006). Unfortunately, national land cover maps generated from satellite imagery are often generalized and not suitable for applications at local levels (Matsika 2007). Good examples include the South African national land cover surveys conducted in 1994 and 2000, which were mapped at scales of 1:250 000 and 1:50 000 respectively (Thompson 1999; Thompson et al. 2001). Although these maps are sufficient for regional (i.e. provincial and district) planning, more detailed (1:10 000 or larger scale) land cover maps are needed for applications at local (i.e. municipal) level.
The absence of detailed land cover maps of South Africa can be partly attributed to the high cost of high-resolution satellite imagery (Lennartz & Congalton 2004) and the large computer processing capacity needed to analyse these data (Hay et al. 1997). The most inhibiting factor is, however, the cost of collecting empirical data to “train” the image classifiers. Traditional inductive techniques such as supervised classification require large quantities of training data to statistically direct the mapping process (Gegg et al. 1990; Carvalho et al. 2004; Inglada 2007). Several representative training areas are needed for each land cover class and, because reflectance properties often vary over distance, sample areas are in many cases required to be collected over the entire region that is being mapped (Campbell 2006). The cost associated with collecting these data often makes national land cover surveys unfeasible.
Rule-based classification offers a possible solution to the high cost of national land cover surveys as it uses expert-informed rules specified in logical structures to identify land cover from remotely sensed imagery and ancillary information (Brown de Colstoun et al. 2003; Kidane 2005). Rule-based classification is therefore less reliant on empirical data obtained though expensive fields surveys and is consequently more cost-effective for mapping large areas (Maxwell et al. 2004). Another advantage of rule-based classification is the logical, flexible and transparent manner in which image information is represented within a rule-set (Nussbaum & Niemeyer 2007). The modular arrangement of rule-sets also allows for easy alterations or updates to improve classification results (Bolstad & Lillisand 1991).
Possibly the biggest advantage of rule-based classification is the ease in which it can be automated using expert systems. Expert systems are computer systems that emulate human decision making (often called artificial intelligence) by considering a set of predefined rules. The main components of an expert system are a knowledge base, which stores information in the form of rules, and an inference engine that contains protocols about how the rules in the knowledge base are applied. A third component, namely a spatial database, is required for spatial applications such as land cover mapping (Van Niekerk 2008). The use of expert systems to automate land cover mapping not only saves time and costs but also reduces the likelihood of human error and subjectivity (Rogan et al. 2008). Although it has been shown that knowledge-based land cover classification is in many respects superior to conventional inductive methods, no research has been done to investigate how it can be used to cost-effectively map detailed land cover in South African on a regular basis.
A major consideration in any land cover mapping exercise is the availability and cost of suitable satellite imagery. Fortunately, since October 2006, SPOT 5 imagery of South Africa can be obtained from the South African National Space Agency (SANSA) at very little or no cost to government and educational institutions. Owing to its relatively high spatial resolution (2.5m), SPOT 5 imagery is well-suited for detailed land cover mapping. The combination of multi-temporal, medium-resolution satellite imagery with a higher spectral resolution (e.g. Landsat 8) will, however, improve classification accuracy (Zhang & Zhang 2007). Further improvements might be effected when ancillary data is used in the classification process (Xian et al. 2009; Zhou et al. (In press)).
The CGA is carrying out research to find the optimal and most efficient methodology to combine and analyse these data sets. The aim is to develop an expert system to cost-effectively produce detailed land cover maps of South Africa on a regular basis using a combination of satellite imagery and ancillary data. To this aim the CGA and students from the Department of Geography & Environmental Studies have been involved in various land cover mapping research projects.
A selection of related projects is listed below.
2014
- Land cover mapping in seven areas of the Eastern Cape (Willowmore, Steytlerville, Port Elizabeth, Grahamstown, Bisho, Butterworth and Flagstaff), for CD:NGI.
2013
- Land cover and vegetation mapping in northern Kimberley, Western Australia (Curtin University, Perth)
- Monitoring salt accumulation and water logging in irrigated areas using remote sensing (Agricultural Research Council)
- Land audit for Matzikama Local Municipality (Department of Rural Development and Land Reform)
2012
- Mapping of urban residential buildings for estimating population growth (ESKOM)
2011
- Land-cover mapping and GIS database development of Saldanha to Cape Town gas pipeline (Chevron and Lyners Consulting Engineers)
2010-2013
- Growth potential of towns in the Western Cape (Department of Environmental Affairs and Development Planning)
2011
- Vegetation mapping and biomass estimation around for Ulco mine (MBB Consulting Services South)
- Land ownership, land-cover, and land-use mapping and change analyses of Stellenbosch municipality (Netherlands Government)
- Berg River catchment land-cover maps of 1987, 1997, and 2007 (Geography & Environmental Studies, SU)
- Land-cover mapping for biomass estimation in Kapau region (Zambia) (Peace Parks Foundation)
2010
- Richards Bay municipality land-cover maps of 1984 and 2003 (Geography & Environmental Studies, SU)
- Land-cover mapping of the Swellendam area (Megan Anderson Landscape Architects)
- Aerial photography (1938) orthorectification (Council for GeoScience)
2009
- Swaziland land-cover map (SSI Environmental Engineers)
- Forest and plantation mapping in Richards Bay area (Geography & Environmental Studies, SU)
2008
- Mapping informal dwellings in Khayalitsha using remote sensing (Psychology, SU)
- Backyard shack survey for Drakenstein Local Municipality (Lyners Consultation Engineers)
- GIS database status and requirements of tourism infrastructure in the Western Cape Province for (Cape Town Routes Unlimited).
- Biomass estimation in the Winelands district (Agricultural Economics, SU)
- Development of the Cape Land-use Expert System (CLUES) for the Western Cape (Geography & Environmental Studies, SU)
Research conducted by the CGA and associates
The CGA is carrying out research to find the optimal and most efficient methodology to combine and analyse these data sets. The aim is to develop an expert system to cost-effectively produce detailed land cover maps of South Africa on a regular basis using a combination of satellite imagery and ancillary data. To this aim the CGA and students from the Department of Geography & Environmental Studies have been involved in various land cover mapping research projects. A selection of related projects is listed below.
In addition to the above projects, the team also has an extensive publication record relating to land cover mapping. Some key papers include (CGA staff members underlined):
- Fourie C, Van Niekerk A & Mucina L 2012. Semi-automated segment generation for geographic novelty detection using edge and area metrics. South African Journal of Geomatics. 1(2): 133-148.
- Fourie CE, Van Niekerk A & Mucina L 2011. Optimising a one-class SVM for geographic object-based novelty detection. AfricaGEO 2011, Cape Town, South Africa. 31 May – 2 June 2011. pp: 1-25
- Mucina L, Daniel G, Stephenson G, Boonzaaier I, Van Niekerk A, Barrett M, Barrett R, Tichý L & Valachovič M 2013. Floristic-Ecological Mapping in the Northern Kimberley: Field Survey Methods and Mapping Protocols. In: Mucina L &
- Daniel G (eds), Vegetation Mapping in the Northern Kimberley, Western Australia. pp. 26-87. Curtin University, Perth, WA. http://academic.sun.ac.za/cga/documents/Kim_Map.pdf
- Mucina L, Stephenson G, Daniel G, Van Niekerk A & Boonzaaier I 2013. A Floristic-Ecological Vegetation Map of the Mitchell Plateau Region (Northern Kimberley, Western Australia). In: Mucina L & Daniel G (eds), Vegetation Mapping in the Northern Kimberley, Western Australia. pp. 88-113. Curtin University, Perth, WA. http://academic.sun.ac.za/cga/documents/Kim_Map.pdf
- Mucina L, Stephenson G, Daniel G, Van Niekerk A & Boonzaaier I 2013. A Floristic-Ecological Vegetation Map of the Pantijan Area (Northern Kimberley, Western Australia). In: Mucina L & Daniel G (eds), Vegetation Mapping in the Northern Kimberley, Western Australia. pp. 114-131. Curtin University, Perth, WA. http://academic.sun.ac.za/cga/documents/Kim_Map.pdf
- Musakwa W & Van Niekerk A 2013. Implications of land use change for the sustainability of urban areas: A case study of Stellenbosch, South Africa. Cities, 33: 143-156. http://dx.doi.org/10.1016/j.cities.2013.01.004
- Myburgh G & Van Niekerk A 2013. Effect of feature dimensionality on object-based land cover classification: A comparison of three classifiers. South African Journal of Geomatics, 2(1): 13-27.
- Myburgh G & Van Niekerk A 2014. Impact of training set size on object-based land cover classification: A comparison of three classifiers. International Journal of Applied Geospatial Research. 5(3)
- Pauw, T & Van Niekerk, A 2012. Automated wetland classification using OBIA: Agulhas plain, South Africa. In Proceedings of the 2012 IEEE International Geoscience and Remote Sensing Symposium, 21-27 July, Munich, Germany.
- Stephenson GR & Van Niekerk A 2009. A cost-effective, rule-based technique to improve forestry inventory on a national scale. In Proceedings of the 2009 IEEE International Geoscience and Remote Sensing Symposium, 12-17 July, Cape Town, South Africa. (ISBN: 978-1-4244-3395-7). pp: III: 459-462. http://dx.doi.org/10.1109/IGARSS.2009.5418077
- Stuckenberg T, Munch Z & Van Niekerk A 2013. Multi-temporal remote sensing land-cover change detection for biodiversity assessment in the Berg River catchment. South African Journal of Geomatics, 2(3): 190-205.
References
- Benz UC, Hofmann P, Willhauck G, Lingenfelder I & Heynen M 2004. Multi-resolution, object-oriented fuzzy analysis of remote sensing data for GIS-ready information. ISPRS Journal of Photogrammetry and Remote Sensing 58: 239.
- Blaschke T, Lang S & Moeller M 2005. Object-based analysis of remote sensing data for landscape monitoring: recent developments. Anais XII Simposio Brassileiro de Sesoriamento Remoto, Goiania, Brasil, 16 – 21 abril 2005, INPE, p.2879-2885.
- Bolstad PV & Lillisand TM 1991. Rapid maximum likelihood classification. Photogrammetric Engineering and Remote Sensing 57: 67-74.
- Brown de Colstoun EC, Story MH, Thompson C, Commisso K, Smith TG & Irons JR 2003. National Park vegetation mapping using multitemporal Landsat 7 data and a decision tree classifier. Remote Sensing of Environment 85: 316.
- Campbell JB 2006. Introduction to remote sensing. London: Taylor & Francis.
- Carvalho LMTd, Clevers JGPW, Skidmore AK & Jong SMd 2004. Selection of imagery data and classifiers for mapping Brazilian semideciduous Atlantic forests. International Journal of Applied Earth Observation and Geoinformation 5: 173.
- CSIR 2009. Satellite Applications Centre [online]. Pretoria: CSIR. Available from [Accessed 25 May 2009].
- Culshaw MG, Nathanail CP, Leeks GJL, Alker S, Bridge D, Duffy T, Fowler D, Packman JC, Swetnam R, Wadsworth R & Wyatt B 2006. The role of web-based environmental information in urban planning—the environmental information system for planners. Science of the Total Environment 360: 233– 245.
- Gegg G, Günter O & Riekert W 1990. Knowledge-based processing of remote sensing data: development of the expert system RESEDA. In Manfred E (ed) Advances in spatial information extraction and analysis for remote sensing, 71-80. Bethesda: American society for photogrammetry and remote sensing.
- Hay GJ, Niernann KO & Goodenough DG 1997. Spatial thresholds, image-objects, and upscaling: A multiscale evaluation. Remote Sensing of Environment 62: 1.
- Inglada J 2007. Automatic recognition of man-made objects in high resolution optical remote sensing images by SVM classification of geometric image features. ISPRS Journal of Photogrammetry and Remote Sensing 62: 236.
- Kalogirou S 2002. Expert systems and GIS: An application of land suitability evaluation. Computers, Environment and Urban Systems 26: 89-112.
- Kidane DK 2005. Rule-based land cover classification model: expert system integration of image and non-image spatial data. MSc thesis. Stellenbosch: Stellenbosch University.
- Lee JT, Woddy SJ & Thompson S 2001. Targeting sites for conservation: Using a patch-based ranking scheme to assess conservation potential. Journal of environmental management 61: 367-380.
- Lennartz SP & Congalton RG 2004. Classifying and mapping forest cover types using IKONOS imagery in the Northeastern United States. Proceedings of the ASPRS Annual Conference, Denver, Colorado.
- Lui X, Skidmore AK & Van Oosten H 2002. Integration of classification methods for improvement of land-cover map accuracy. ISPRS Journal of Photogrammetry and Remote Sensing 56: 257-268.
- Matsika R 2007. Land-cover Change: Threats to the Grassland Biome of South Africa. MSc thesis. Johannesburg: University of the Witwatersrand.
- Maxwell SK, Nuckols JR, Ward MH & Hoffer RM 2004. An automated approach to mapping corn from Landsat imagery. Computers and Electronics in Agriculture 43: 43.
- Nussbaum S & Niemeyer I 2007. Automated extraction of change information from multispectral satellite imagery. Esarda, Bulletin 36: 19-25.
- Phua M-H & Minowa M 2005. A GIS-based multi-criteria decision making approach to forest conservation planning at a landscape scale: A case study in the Kinabalu Area, Sabah, Malaysia. Landscape and Urban Planning 71: 207-222.
- Rogan J, Franklin J, Stow D, Miller J, Woodcock C & Roberts D 2008. Mapping land-cover modifications over large areas: A comparison of machine learning algorithms. Remote Sensing of Environment 112: 2272.
- Tapiador FJ & Casanova JL 2003. Land use mapping methodology using remote sensing for the regional planning directives in Segovia, Spain. Landscape and urban planning 62: 103-115.
- Thompson M, Van den Berg HM, Newby TS & Hoare D 2001. Guideline procedures for national land-cover mapping and change monitoring. Report No. ENV/P/C 2001-006. Pretoria: CSIR/ARC.
- Thompson MW 1999. South African national land-cover database project: data users manual. Pretoria: CSIR.
- Van Niekerk A 2008. CLUES: A web-based land use expert system for the Western Cape. PhD dissertation. Stellenbosch: Stellenbosch University.
- Xian G, Homer C & Fry J 2009. Updating the 2001 National Land Cover Database land cover classification to 2006 by using Landsat imagery change detection methods. Remote Sensing of Environment 113: 1133.
- Zhang J & Zhang Y 2007. Remote sensing research issues of the National Land Use Change Program of China. ISPRS Journal of Photogrammetry and Remote Sensing 62: 461.
- Zhou W, Huang G, Troy A & Cadenasso ML (In press). Object-based land cover classification of shaded areas in high spatial resolution imagery of urban areas: A comparison study. Remote Sensing of Environment In Press, Corrected Proof.